From LLMs to seismic foundation models, AI can be utilized for subsurface E&P
By Wadii El Karkouri, TGS
Large language models (LLMs) are driving a significant shift across industries, offering new levels of efficiency, cost savings and data-driven insights. Initially developed to process and generate human language, LLMs have quickly gained recognition for their ability to handle vast amounts of text. However, their adaptable architecture is now being applied in various fields. From healthcare and finance to manufacturing, LLMs automate workflows, improve decision-making and uncover deeper insights from complex datasets.
The impact of LLMs in the energy industry is particularly promising. As the sector faces growing demands for enhanced operational efficiency, lower costs and more accurate subsurface data, LLMs are being tailored to solve challenges specific to exploration and production (E&P). With their ability to process and analyze vast data efficiently, LLMs are helping energy companies streamline operations, make more informed decisions and drive innovation, particularly in subsurface geology and resource management.
LLMs for subsurface applications
LLMs have revolutionized fields, like natural language processing, by learning from massive datasets, making them adaptable for various tasks with minimal additional training. When applied to subsurface exploration, LLMs have the potential to streamline data interpretation and automate decision-making processes that previously required manual intervention.
One of the most exciting applications of LLMs in subsurface exploration is their ability to handle domain-specific text data, such as technical reports and operational documents, that geoscientists and engineers rely on for decision-making. LLMs can automatically summarize lengthy technical documents, extract key insights and even recommend best practices based on historical data.
However, while LLMs are effective for textual data, subsurface exploration often requires processing diverse data types, including seismic volumes, well logs and geological maps. This is where the power of multimodal machine learning models becomes essential.
Role of multimodal learning
Multimodal learning refers to models that can process and integrate different types of data, also called modalities. In subsurface exploration, multimodal models can analyze data from various sources (e.g., seismic surveys, well logs, core samples and production data) all at once. This capability allows them to generate a more comprehensive understanding of the subsurface environment.
While LLMs excel in handling textual data, multimodal models are more adept at correlating multiple input types to provide actionable insights. For example, a multimodal model can combine seismic data with geological maps to identify hydrocarbon reservoirs or predict drilling hazards by cross-referencing past drilling logs and seismic interpretations.
By leveraging multimodal learning, these models can provide holistic subsurface insights beyond what can be inferred from a single data type. This is critical in complex environments, such as offshore basins, where decision-making relies on understanding the interplay between geophysical datasets.
Seismic foundation models
TGS has adapted the principles of multimodal computer vision models to create seismic foundation models specifically designed to handle seismic data and, in the future, other geophysical inputs. Seismic foundation models build upon the foundation laid by LLMs and multimodal models. Still, they are tailored to meet the unique demands of subsurface exploration, particularly in interpretation tasks and processing seismic data.
Seismic foundation models are pre-trained on vast global seismic datasets collected from multiple basins. This large-scale pre-training enables the models to generalize effectively across various geological formations and regions, providing valuable exploration, drilling and reservoir management insights.
Building a seismic foundation model is all about scale, and TGS leads this development with an extensive corpus of multiclient seismic data. By utilizing a cloud-based data management ecosystem, optimized I/O modules and the chunked seismic data format (MDIO), TGS maximizes GPU utilization during training, ensuring a highly efficient and scalable process.
Looking ahead
As TGS continues to develop its seismic foundation models, the potential for integrating even more data modalities grows. Combining seismic data, well logs, natural language and production data into a single model will allow TGS’ multimodal models to deliver even more accurate and comprehensive insights into subsurface conditions. Partnerships with cloud providers and AI leaders will enable the scaling of these models, ensuring they remain indispensable tools for the offshore oil and gas industry.
About the Author
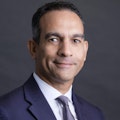
Wadii El Karkouri
Wadii El Karkouri is the executive vice president of Imaging & Technology at TGS.
With more than 25 years of leadership experience in the energy and technology industries, he drives innovation and technological advancements in seismic processing, data analytics and digitalization across the company.
Prior to joining TGS, he served in a range of leadership roles with SLB in the US, Africa, Asia, Europe and the Middle East, including global vice president of WesternGeco Geosolutions and global vice president of WesternGeco Sales & Commercial, as well as managing executive relationships with global energy companies at AWS as a global sales director of AWS Energy and Utilities.
He is a Moroccan citizen and currently resides in Houston, Texas.